Sequoia: Scalable, Robust, and Hardware-aware Speculative Decoding
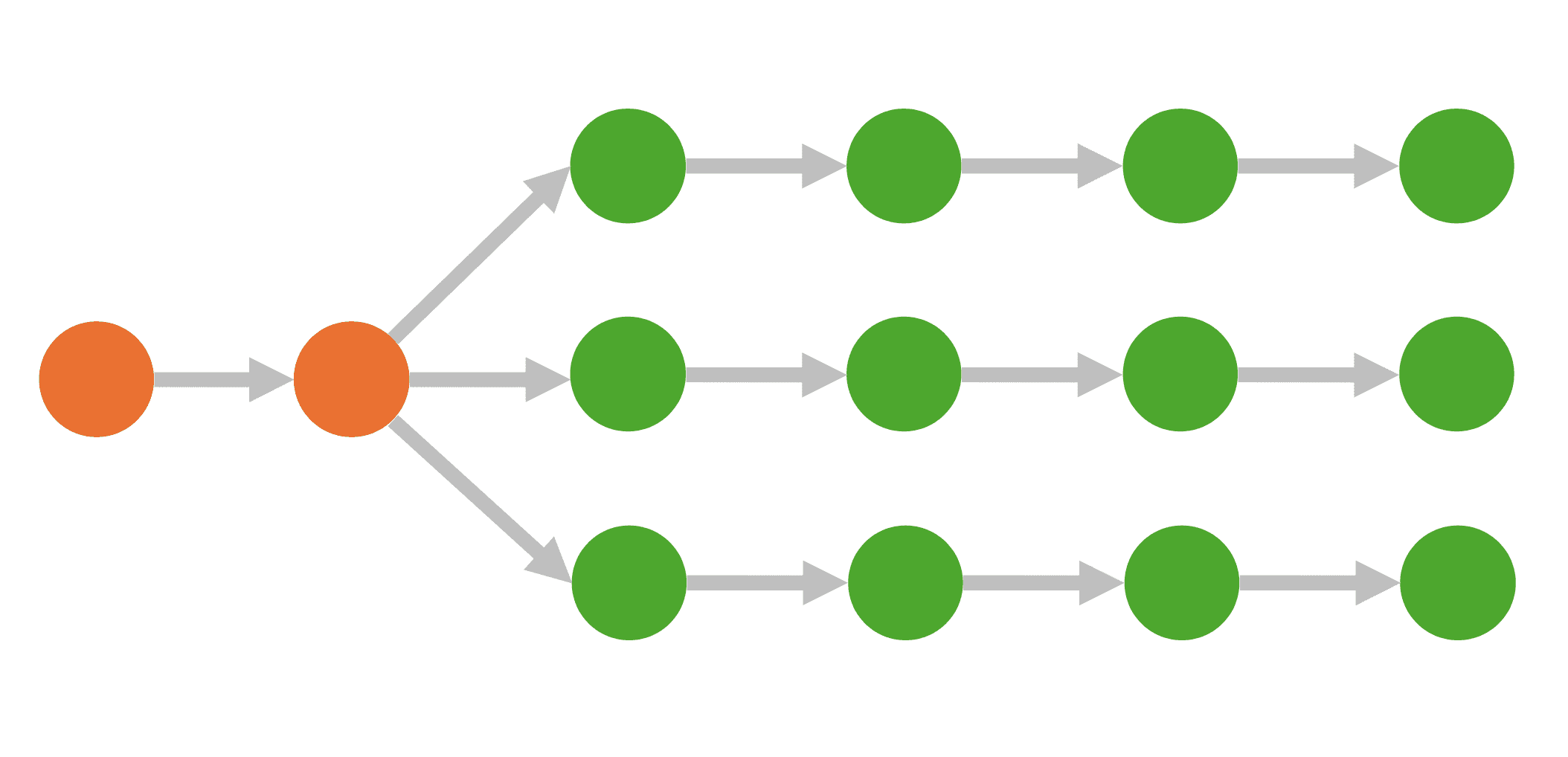
As the usage of large language models (LLMs) grows, it becomes increasingly important to serve them quickly and efficiently. While speculative decoding has recently emerged as a promising direction for accelerating LLM serving, existing methods are limited in their ability to scale to larger speculation budgets and adapt to different hyperparameters. This paper introduces Sequoia, a scalable and robust algorithm for speculative decoding. To improve scalability, Sequoia introduces a dynamic programming algorithm to find an optimal tree structure for the speculated tokens. To achieve robust speculative decoding, Sequoia uses a novel sampling and verification method that outperforms prior work across different decoding temperatures. Sequoia improves the decoding speed of Llama2-7B, Llama2-13B, and Vicuna-33B on an A100 GPU by up to 4.04×, 3.73×, and 2.27×. To serve Llama3-70B-Instruct on a single L40 GPU through offloading, Sequoia reduces the per-token decoding latency to 0.60 s/token, 9.5× faster than DeepSpeed-Zero-Inference.